Is AI Really Intelligent? The Generative AI Paradox
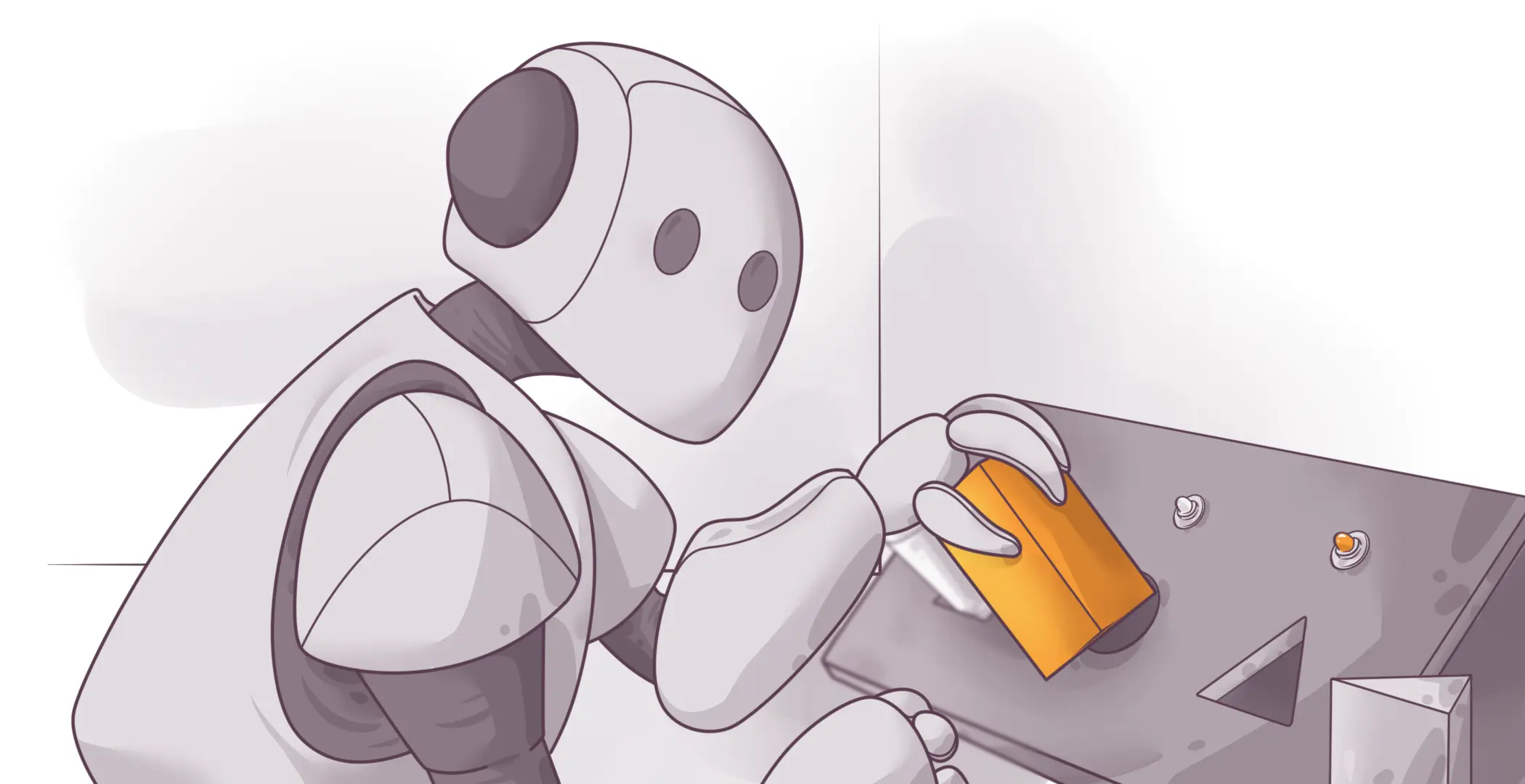
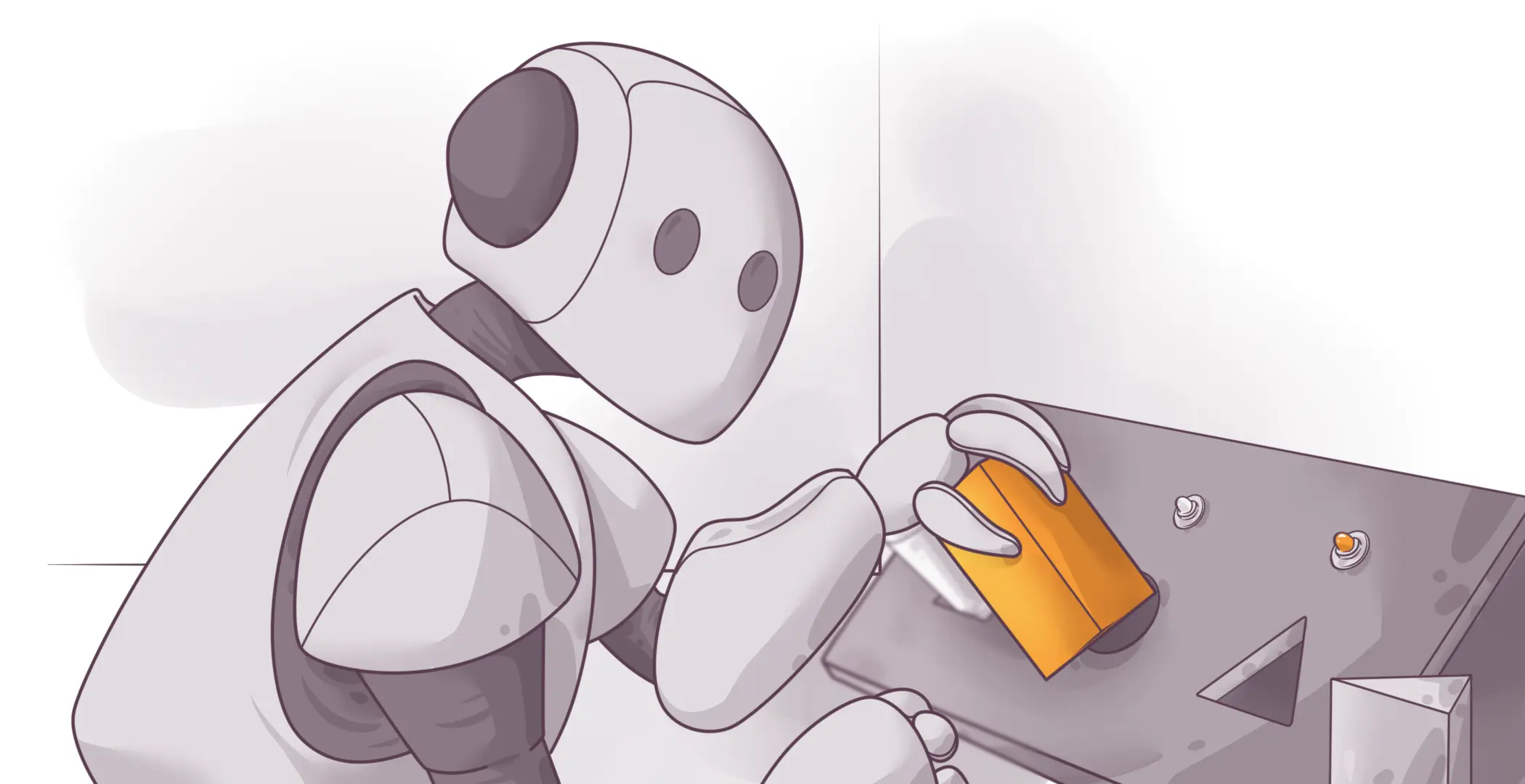
Some time ago, a new paper appeared about, let's say, how intelligent the new LLM models like GPT-4 are. And when you read this document, you learn that AI is not so intelligent, or maybe it's not intelligent at all. Is that so?
This paper is called "THE GENERATIVE AI PARADOX: ‘What It Can Create, It May Not Understand’". Here it is.
Let's dive into the Generative AI Paradox and understand how these researchers determined that humans are smarter than the GPT-4 model, for example.
To prepare this paper, the authors had a crucial question: Do these AI models truly understand what they create? This question forms the crux of the Generative AI Paradox.
To understand all this, let's first understand two concepts about two different tasks that a model can perform:
- Generative tasks are those that involve creating new content, like writing a story or designing an image. This is where AI models particularly excel. So, every time we talk about Generative tasks, we are talking about something that the AI is going to create for us.
- In Discriminative tasks, the model has to choose from predefined options or categorize data into existing groups. For example, in natural language processing, a generative task might be to write a story. In contrast, a discriminative task could be classifying a text as positive or negative, or selecting the correct answer from a set of options in a reading comprehension test.
The Generative AI paradox comes from an interesting observation: AI models are really good at creating detailed content similar to what experts do, but they often make basic mistakes in understanding that we wouldn't expect, even from people who are not experts. To explore this further, we use two types of evaluations: Selective Evaluation and Interrogative Evaluation.
Selective Evaluation: This evaluation assesses whether models can choose the correct answers from a set of options, testing their ability to understand and distinguish between different choices. It's a key part of seeing how practical and effective AI applications are.
Imagine an AI model is given a task to read a short story and then answer a multiple-choice question about it. The question might be: "What is the main theme of the story?" with four options provided: A) Friendship, B) Adventure, C) Love, and D) Betrayal. The AI's task is to read the story, understand its main theme, and select the correct option from the given choices.
Interrogative Evaluation: In this evaluation, we challenge models by asking them questions about the content they have created. This is a direct way to see if AI really understands its own creations. It helps us understand the depth of AI's comprehension and its ability to reflect on what it has generated.
For this, let's say the AI model generates a story about a young girl who overcomes her fears and wins a swimming competition. After generating this story, the AI is asked: "Why was the swimming competition important to the main character?" The AI must understand its own narrative to provide a coherent answer, such as "The competition was important to her because it was a way to overcome her fears and prove her strength." This tests the AI's ability to comprehend and explain the content it generated.
In this paper, a large number of experiments were conducted in both language and vision modalities to test these hypotheses with one question in mind: Does the AI truly understand its creations? These ranged from generating texts and creating images to answering questions about these creations.
After all those tests, they got a result in the two kinds of evaluations that we saw before:
In Selective Evaluation, models often outperformed humans in content generation but were less adept at discrimination and comprehension tasks.
In Interrogative Evaluation, models frequently failed to answer questions about their own creations, highlighting a disconnect between generation and comprehension.
So, the AI is not able to understand what it creates, and the reason for that is because it is trained to generate after training and not to understand what was generated. It's like a machine creating a toy one after the other, day by day. It can create something but not understand what that is.
These findings challenge our preconceived notions of AI. Although models can mimic human creativity, their understanding of content remains superficial.
This study is really important and helps start more research in the future. Being able to repeat this research and add more to it is key to understanding AI better.
The Generative AI Paradox gets us to think differently about how smart machines really are. Even though they can create a lot of things, AI still needs to learn a lot about truly understanding.
Here' the same article in video form for your convenience: